Machine Learning in Automotive Systems
The Power Of Machine Learning In The Automotive Sector
Artificial Intelligence (AI) has assumed the centre stage of world affairs in today’s technically advanced world. Also, there has been quite a lot of commonplace talk regarding self-driving cars. Overhyped though the concept of self-driving cars is so far as practicality is concerned, technological innovations in that direction are noteworthy. But hey! Did you just think artificial intelligence is the same as machine learning just because we started our discussion with it?
Difference between Machine Learning and Artificial Intelligence
Artificial intelligence is the ability of a computer system to effectively emulate the cognitive capabilities of a human being such as learning and problem-solving.
Machine learning is a subset of artificial intelligence that is concerned with designing and developing algorithms that manipulate and analyse data. Machine learning algorithms find applications in in-vehicle navigation, fraud detection, and consumer recommendations.
In a way, they are interconnected because machine learning facilitates Artificial Intelligence in a computer system.

A Sneak Peek into how Artificial Intelligence and Machine Learning are Intertwined
Firstly, an AI system is built using machine learning and other associated procedures.
What follows is the creation of machine learning models by studying patterns in the data.
Next, data scientists optimise the machine learning models relying on data patterns.
The aforementioned chronology is repeated for accuracy.
A heavy dosage of computer science stuff having been administered, we are now all set to learn about the most interesting application of machine learning.
The Potential Of Machine Learning’s Usage In The Automotive Sector
From improving safety to reducing emissions- machine learning has a vast spectrum of applications in the automotive field. We will be discussing some of them in detail.
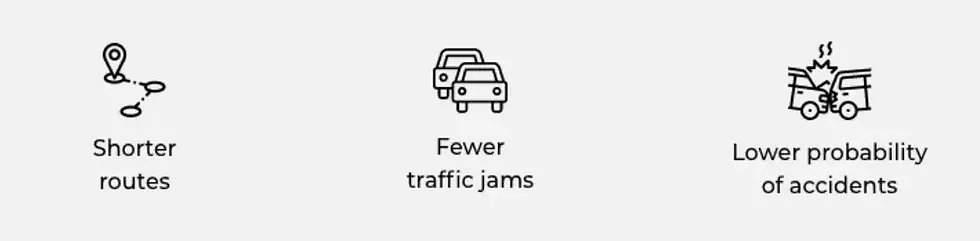
1. Enhancing Safety
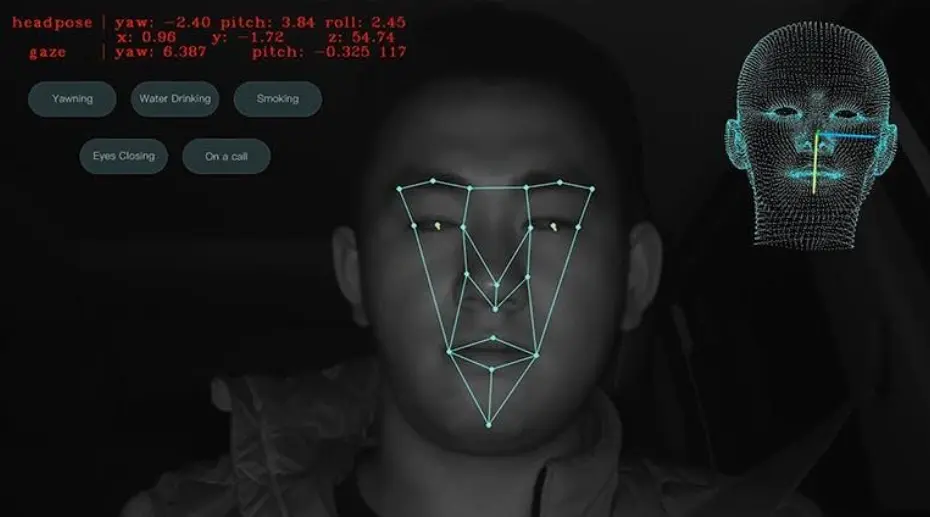
Machine learning can be used to formulate advanced driver assistance systems that can aid accident prevention.
These systems serve to identify potential hazards and warn drivers of potential danger by analysing data from sensors and cameras. Let’s now learn about the ADAS HOT feature in automobiles being sold these days.
2. Advanced Driver Assistant System (ADAS)
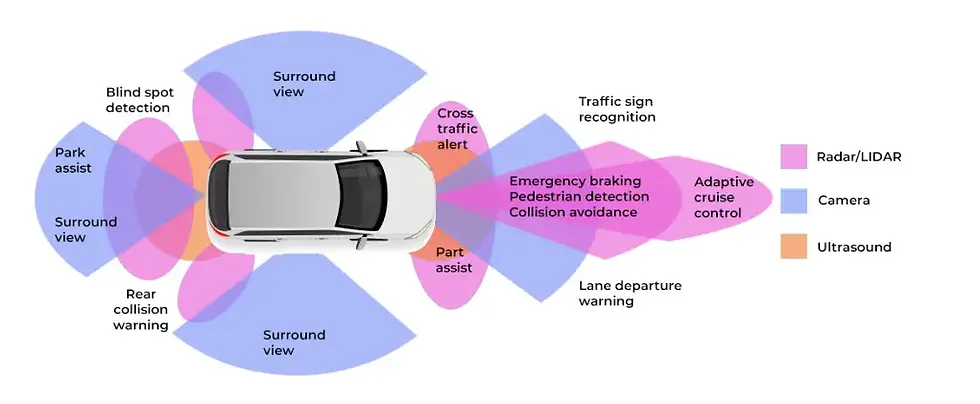
With ADAS, driving becomes more foreseeable. Every driver has a unique style of driving – the reason why there is so much confusion on the road regarding the next actions of fellow drivers. ADAS standardizes a safer approach to driving. ADAS can make decisions faster, and provide better solutions faster than humans are capable of doing so. ADAS can also take care of blind spots, pulling the emergency brake as and when the situation demands.
3. Reducing Emissions
Machine learning algorithms can figure out ways to optimize routes and abate fuel consumption by analysing data on driving patterns. This can contribute significantly to making the vehicle more fuel-efficient and cost-effective.
4. Automated Driving Systems
Some reputed brands like Tesla, Mercedes, and BMW are testing and building vehicles with automated driving systems (ADS). While real self-driving is yet to become a reality, we like to refer to these as autonomous vehicles or self-driving cars – which is true in certain aspects. Vehicles equipped with ADS are already able to handle manoeuvres like parking with full autonomy and should be able to operate entirely without a human in the future.
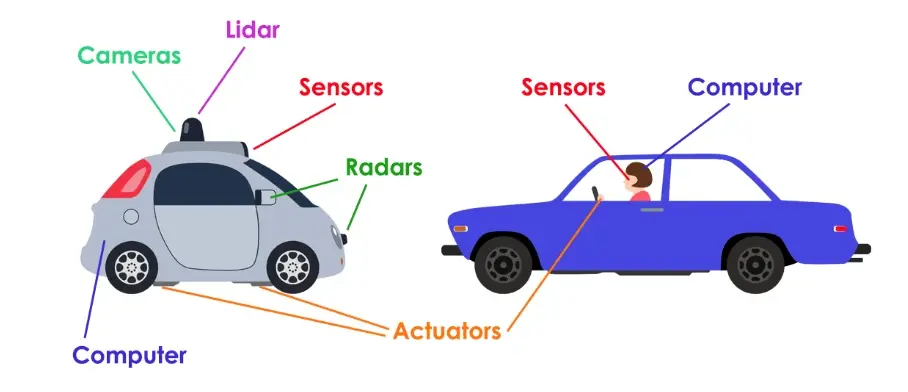
Self-driving cars operate using three major types of sensors, namely cameras, lidar, and radar systems. These systems work together just like our brain, eyes, and ears do to perceive the environment. Machine learning algorithms trained on real-life data sets. A car equipped with machine learning has two types of learning models: supervised and unsupervised. The unsupervised learning model means that the algorithm receives unlabelled data and has no predefined instructions on how to process it, so it needs to figure it out on its own. With the supervised learning model, the algorithm gets fed with instructions on how to label the data. For cars with ADS, supervised learning is mostly opted for.
Electronic components that facilitate self-driving cars
The aforesaid topic, if I may take the liberty to assume, has struck you as interesting, hasn’t it? So let’s have a quick peek into the electronic components that facilitate self-driving cars.
1. Imaging Radar
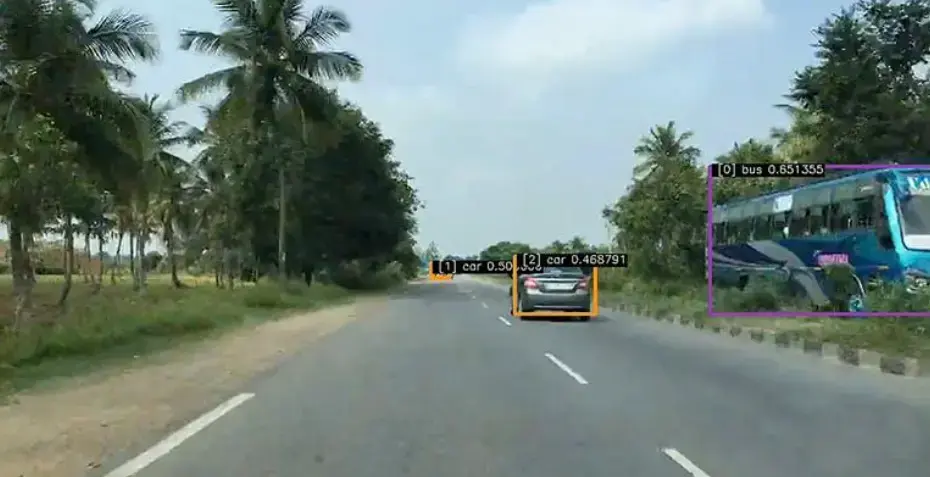
Imaging radar is a high-resolution radar that can both detect as well as classify objects. Apart from its basic capabilities as a radar, imaging radar offers greater density in the reflected points that it accumulates. So, not only does it detect an object and work out its proximity, but it also uses the collection of all the points to start outlining the objects that it is picking up. From those outlines, it is possible to begin to make decisions about the classification of the object that’s being reflected.
2. Compute Performance
Training is the central aspect of machine learning. To reach anywhere close to human capabilities and avert the risk of anomalies, the training required needs continual exposure of the system to the varied and less common situations that occur on urban roads, highways, and freeways.
As an increasing track of road miles is gathered by car manufacturers, and more objects require detection and classification, the data sets being created get augmented.
3. LiDAR
Another rising trend in machine learning for the automotive market is to transfer the procedures currently being used for camera-based classification and detection over to LiDAR networks. So, instead of using a two-dimensional picture frame to determine and classify objects, it’s possible to use three-dimensional data obtained from LiDAR reflections and then implement trained networks on that data. In this way, the system can determine details such as where the road begins and ends; the presence of the cross junctions; or the location of the traffic signal posts.
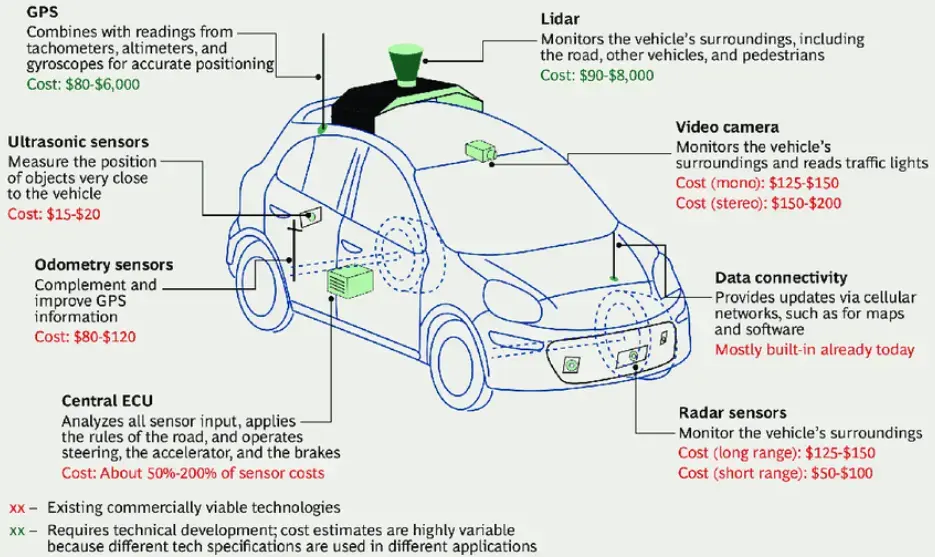
This has been possible using convolutional neural networks (CNNs), which is a class of deep learning. Companies are already working on this technology—with success—and it’s an area that’s showing great promise.
4. Fully Integrated Microcontroller Units
Fully integrated microcontroller units (MCUs) will enable the next generation of autonomous vehicles. At Level 5, MCUs will enable the vehicle to detect a fault, and then automatically bring the car safely to a halt without any intervention from the driver.
Current MCUs are obtained from graphics or desktop and enterprise computing. The MCU sits alongside the processor and interacts with the vehicle.
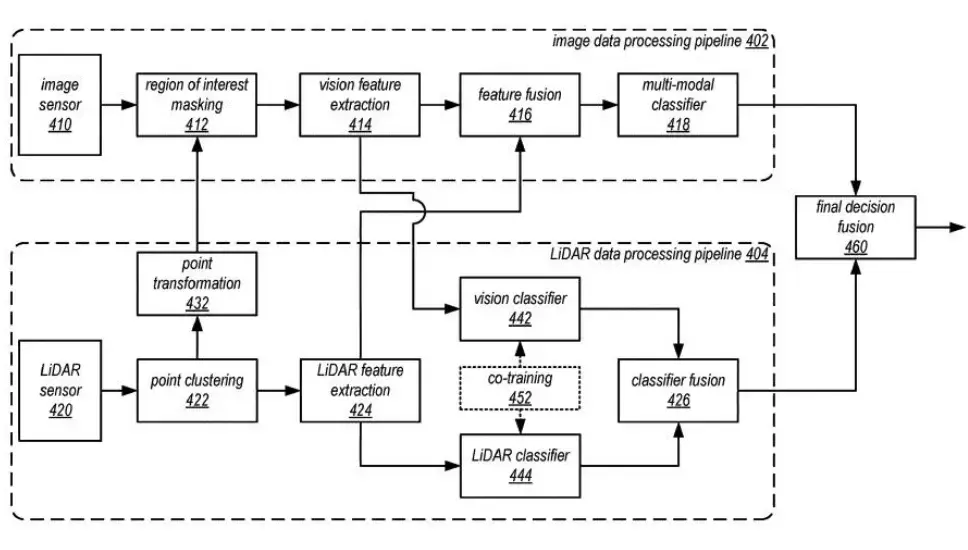
The MCU can run safety self-checks on the high-performance integrated circuit, with diagnostic capabilities that make sure the system-on-chip (SoC) is healthy and performing optimally. The MCU acts as a core processor to combat the inherent deficiencies that even reside in high-performance processors because they’re not designed keeping the automotive environment in mind.
5. Predictive Maintenance
Machine learning can assist in protecting the safety of both drivers and vehicles by providing accurate as well as up-to-date maintenance recommendations. A vehicle with predictive maintenance collects data all through the day, and alerts based on anomalies, data analytics can predict or ascertain when the vehicle requires maintenance, as a result averting breakdowns and expensive repairs.
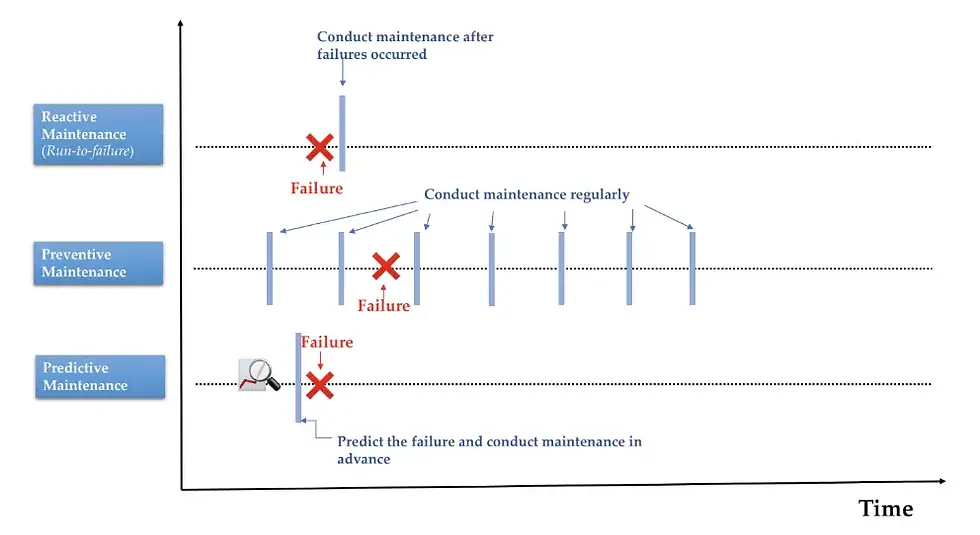
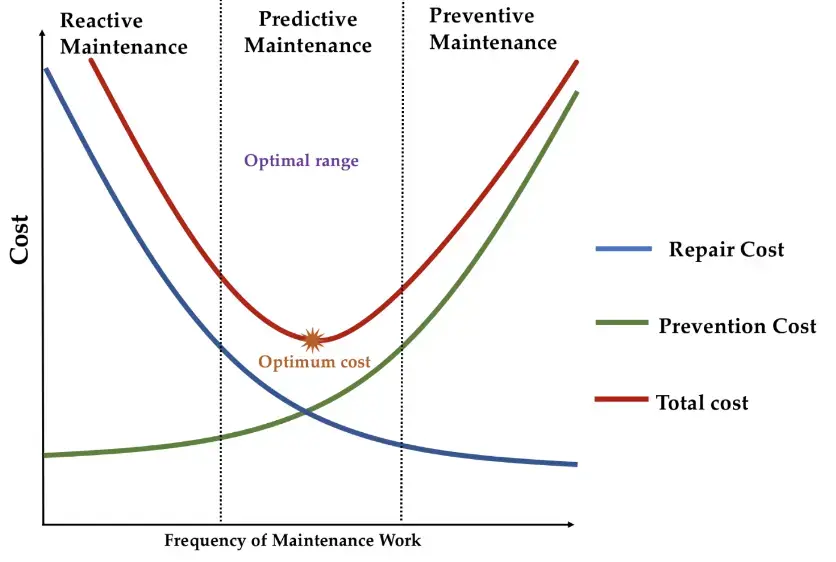
6. Quality Control
Quality is one of the topmost concerns in the automotive industry. Enforcing quality control throughout the assembly line is imperative – and machine learning helps do this at optimal costs. The use of ML can help lower manufacturing costs through quality enhancement and waste reduction. Machine learning can analyse and learn which aspects are fundamental and frame rules that decide the combination of tweaks and features that define the quality of products. It can also appreciate the implicit relationship between large data sets in complex and dynamic environments. The quality of every component can be a crucial factor in a critical life-and-death situation. Using sensor-based artificial intelligence, defective objects are immediately eliminated from the production line. The use of AI can help reduce the percentage of defective products released, implying safer cars on our roads.
7. Supply Chain optimization
Supply chain optimization is a tedious task for any type of industry, with the automotive industry being no exception due to the ever-increasing complexity of both its end products and the manufacturing processes it relies on. It makes the maximum possible use of technology and resources like blockchain, AI, and IoT to enhance efficiency and performance in a supply chain. Machine learning enables the software to learn and adjust without it being explicitly programmed to do so.
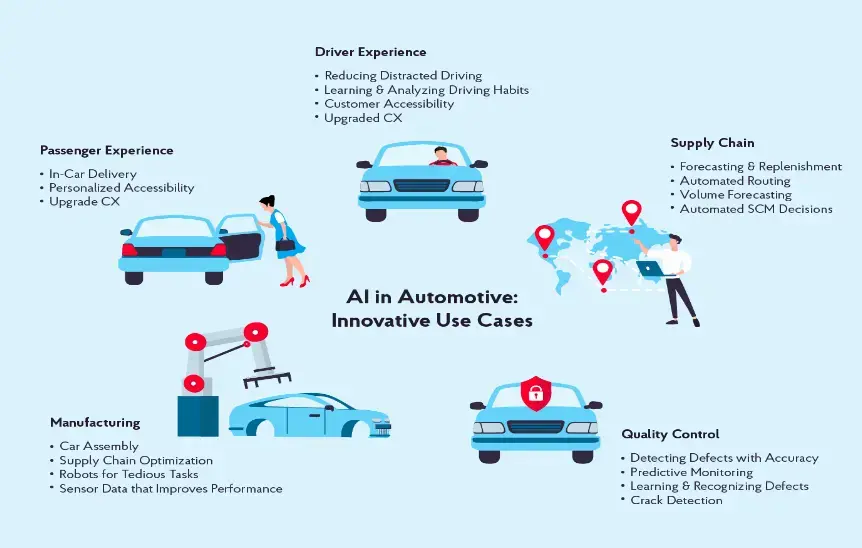
Nowadays, supply chains generate a huge amount of complex data. ML can analyse this information and use it to optimize supply chain management. It can also analyse timings and handovers as products proceed through the supply chain. After analysing the accumulated data, it can identify potential bottlenecks and suggest checks to optimize the supply chain. Using machine learning, organizations in the supply chain don’t need to depend on inventory as much, since ML helps optimize the flow of the product from one stage to another. This greatly encourages manufacturing efficiency, regardless of whether the carmaker decides to keep inventory due to other reasons (such as shortages, which we’ve encountered a lot of in recent years).
Benefits Of Using Machine Learning In The Automotive Industry
Machine learning can potentially make the automotive industry environment-friendly and more efficient. For example, it can help increase the usage of alternative fuels by enabling vehicles to run on multiple fuel types or make transitions seamlessly between fuels based on their relative costs. It can also allow vehicles to become more energy-efficient while in motion by regulating factors such as aerodynamics and engine power via machine learning algorithms.
Drawbacks Of Using Machine Learning In The Automotive Industry
One of the most formidable challenges in using machine learning in the automotive industry is the requirement for large amounts of data. Machine learning algorithms need a large dataset to learn and make predictions. The automotive industry does produce a large amount of data, but it is often siloed within individual companies. It isn’t easy to obtain the quintessential data for training machine learning models.
Another challenge is that the automotive industry is constantly evolving. New technologies and regulations are brought forth every year. This makes it difficult to keep machine learning models up-to-date. Automotive companies need to invest a large chunk of their resources into maintaining their machine-learning models.
Also, machine learning models can be challenging to interpret. Automotive decision-makers need to understand how the models work to make sound decisions.
Despite these challenges, machine learning is still an important tool for the automotive industry. Automotive companies that integrate machine learning into their software development can gain an edge over other competitors.
Future of Machine Learning in the Automotive Industry
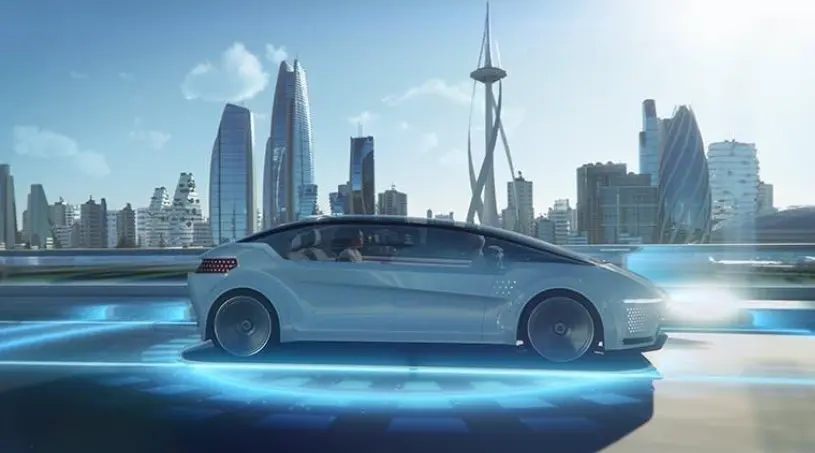
There are already several existing companies in the automotive sector that are using machine learning. For example, Tesla employs machine learning algorithms to improve the performance of its autonomous vehicles. BMW uses machine learning to formulate a system to detect potential accidents and alert drivers. Mercedes-Benz is using machine learning to promote and power its Mercedes Connect service, which provides drivers with information about their car’s status and location. Thus, the automotive sector is just beginning to scratch the surface of what is feasible with machine learning. As the technology continues to develop, we can expect to find even more innovative and transformative applications of machine learning in the automotive sector.
Conclusion
It’s unlikely that we can expect full-scale production models of autonomous vehicles before 2025, and Level 5 cars before 2035. Beyond that, it remains to be seen how long it might take before the number of autonomous cars outstrips those driven manually.
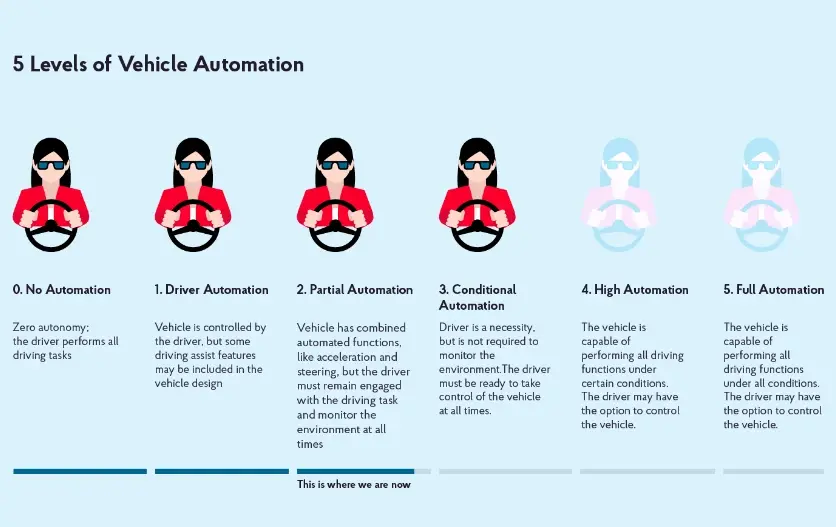
Nevertheless, autonomous cars and trucks are certainly on the horizon. Thanks to ML, these vehicles are set to bring enhanced mobility to millions of vision-impaired and disabled people; enable deliveries in remote areas, getting goods to people more quickly and cost-effectively and connecting communities; and above all, improve road safety, reducing road traffic incidents, injuries, and deaths.
But to transform our lives for the better, some factors still need to come together. Car manufacturers will have to do their part to ascertain the safety, reliability, and viability of these vehicles. They, of course, want a return on their investment in research as well as development, but they will need to prove the safety and security of driverless vehicles before consumers can readily accept them
Governments also have a part to play. They will need to legislate on the autonomy of vehicles and the absence of a driver. It’s a certainty that different nations will take different approaches to this matter. Cooperation and collaboration in this sphere will go a long way toward helping the industry provide standardized vehicles with similar or similar features.
Comments